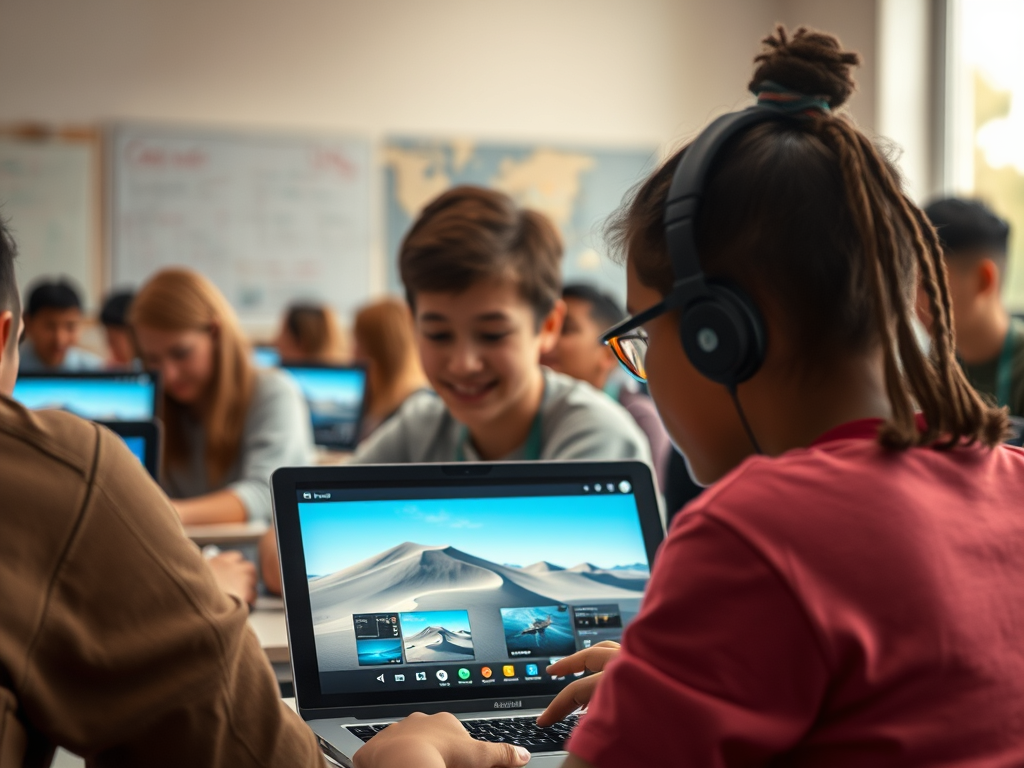
Using Google’s Teachable Machine to Reinforce Academic Vocabulary and Deepen Understanding
There is a legitimate concern that students will use artificial intelligence as a shortcut, that their critical thinking skills will be negatively affected by using AI. Conversely, safe and ethical AI use requires critical thinking and media literacy. AI systems have been known to hallucinate information, make up sources, and struggle with nuances of language. AI systems only know what we feed them. They rely entirely on data generated by humans.
Rather than teaching students to access information on AI, we can reverse the process and have students themselves teach an AI machine. Students can feed AI models data to teach it to understand academic vocabulary. In the process, students strengthen their understanding of the terms themselves. This process is possible with Teachable Machine by Google: a free, intuitive tool that helps students train a simple machine learning model using images, sounds, or poses. There is no sign-in required, and there is great potential for cross-curricular learning.
Learning by Teaching (the Machine)
Students can use Teachable Machine to differentiate between two related concepts such as solid vs. liquid, night vs. day, or triangle vs. quadrilateral. To train the model, students collect or create multiple examples of each concept, categorizing them accordingly. In teaching the AI to recognize the differences between two terms or contexts, students internalize their characteristics. In the image below, students trained a model to identify an image of a desert and a tundra.

But the true deep thinking comes next. Students test the model by providing the system with a new input, asking the AI to categorize an image it has not been trained on. As seen in the image below, small sample sizes often result in miscategorizations by the AI. The model is 100% confident that the image on the left belongs in the category “desert”. However, the AI is also 99% certain that the image on the right should be categorized as “tundra”. As students review the AI’s conclusions, they must consider why the machine made a mistake. This often calls for reviewing data that the machine had been trained on, looking for patterns, and determining what new data needs to be fed to the model to produce a more accurate output.

Steps for Teaching Vocab to a Machine:
- Pick Two Concepts: Choose vocabulary or ideas students are currently learning.
- Collect Examples: Students take photos, make sounds, or strike poses that represent each idea. I have seen this include major vs. minor chords in music, obtuse vs. acute angles in math, and content vs. frustrated faces for social emotional learning.
- Train the Model: Upload the examples into Teachable Machine, label the classes, and click a button to train the model.
- Test & Reflect: Feed a new input into the machine to test if it can accurately distinguish the concepts. Identify reasons why it succeeded or struggled.
- Extend the Thinking: Fuel critical thinking by encouraging students to note their reflections on an adapted Frayer model as seen below.

The Power of Teaching a Machine
This activity supports multimodal learning by encouraging students to move beyond words and use visuals, movement, or sound to define ideas. It can easily be used in science, ELA, math, social studies, or any other content area in which students are exploring key concepts and/or academic vocabulary terms. Along the way, students build AI literacy by gaining hands-on experience with how machines learn and where their limitations lie. The process also promotes metacognition, prompting students to reflect on how they know what they know as they teach the machine to distinguish between ideas.
I have shared this slide deck with teachers to support the process. It includes screenshots demonstrating how to utilize the digital tool and a frayer model template. Feel free to copy and paste for your own use if it is helpful.
When students teach a machine to “understand,” they engage in a meaningful types of learning: constructing knowledge through creativity and comparison. And simultaneously, they build critical digital literacy skills that will serve them far beyond the academic content they are teaching the machine.
Wonder. Explore. Learn. And now—teach the machine.